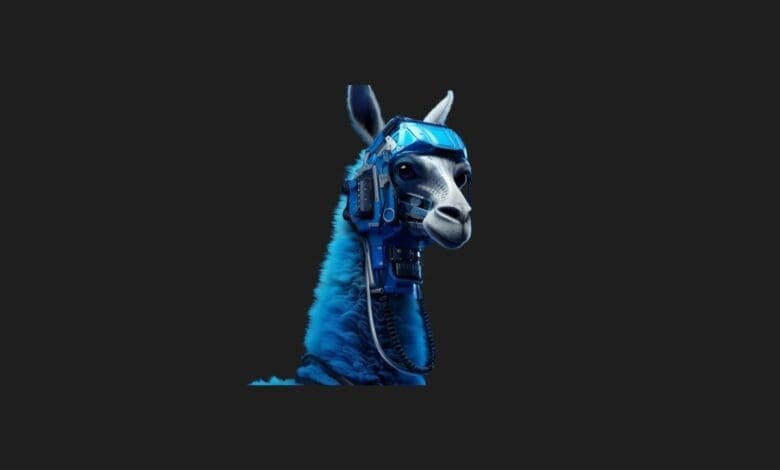
Meta Llama 3 Model Malfunction: GPU Revolt Causes User Upset
The Meta Llama 3 model malfunctioned 419 times in 54 days. Scalability issues, GPU errors, and various other malfunctions led me to give up.
According to Meta’s new research report, the cluster of 16,384 NVIDIA H100 GPUs used to train the 405-billion-parameter Llama 3 model has been problematic. It malfunctioned 419 times in 54 days, averaging one breakdown every three hours.
Meta Llama 3 language model malfunctions every three hours
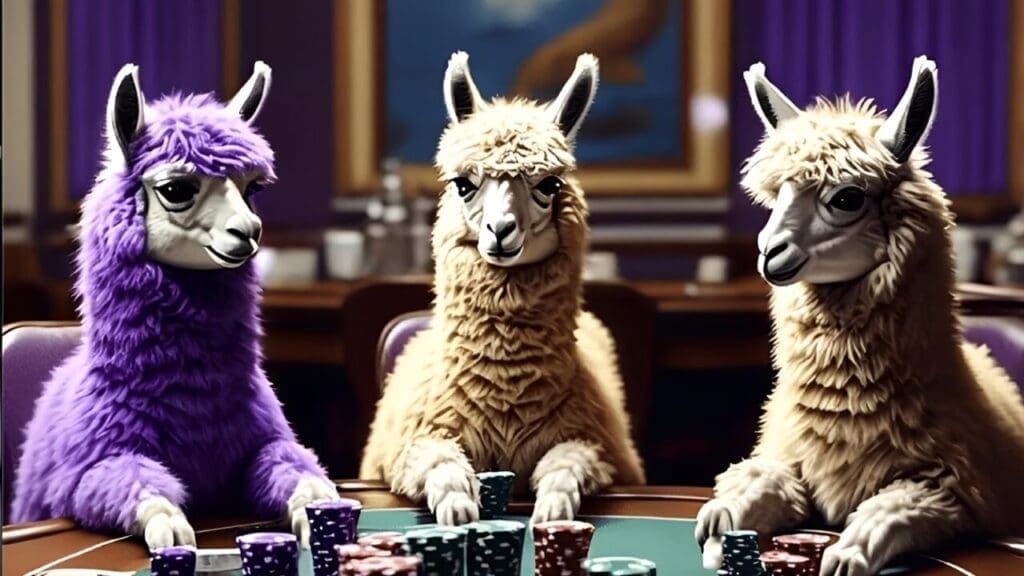
The scale of the Llama 3 language model system and the synchronization of tasks are so precise that even a single GPU failure can halt the entire training process, requiring it to restart. According to the Meta team’s report, of the 419 failures, 148 (30.1%) were due to various GPU issues, while 72 (17.2%) were caused by problems with the GPU’s high-bandwidth memory (HBM3). Remarkably, there were only two CPU failures in those 54 days. The remaining 41.3% of unexpected outages were attributed to software errors, network cables, and adapter issues.
The Meta team has developed a range of tools and strategies to manage these challenges. They implemented measures such as reducing task launch and checkpoint times, using PyTorch’s NCCL flight recorder for diagnosing performance issues, and identifying faulty GPUs. They also considered environmental factors, including the impact of temperature fluctuations on GPU performance and the strain on the data center’s power grid from running numerous GPUs simultaneously.

As the number of parameters in AI models, like the 405-billion-parameter Llama 3, continues to grow, large training clusters will become more common. For instance, the xAI plan’s 100,000 H100 graphics card cluster suggests that future AI training may face even greater challenges. Therefore, Meta’s efforts to address these issues are crucial for the success of larger-scale projects in the future.
Meta has achieved over 90 percent effective training time, though efficiency could have been higher without these failures. These experiences will help Meta build more robust and resilient systems for future projects.
What are your thoughts? Feel free to share your opinions in the comments section below.
You may also like this content
- Meta Building World’s Fastest AI Supercomputer for Metaverse
- Artificial Intelligence Will Make Decisions Instead Of People
- Bill Gates: Artificial Intelligence Over Web3 and Metaverse
Follow us on TWITTER (X) and be instantly informed about the latest developments…