
The Bias in Artificial Intelligence: Unveiling the Truth
Yes, artificial intelligence (AI), and more specifically machine learning (ML) models, can be biased. Here’s why:
Training Data Biases

Machine learning models, which are a subset of AI, learn from data. If the data they are trained on is biased, the models can inherit and even amplify those biases. For instance, if an AI is trained on historical hiring data where certain groups were underrepresented or unfairly treated, it might perpetuate those biases when making hiring recommendations.
Selection Bias

If the data used to train an AI does not accurately represent the broader population or the specific context in which the AI will operate, it can lead to biases. For example, a facial recognition system trained mostly on images of people from one ethnicity may perform poorly on people from other ethnicities.
Design and Model Biases
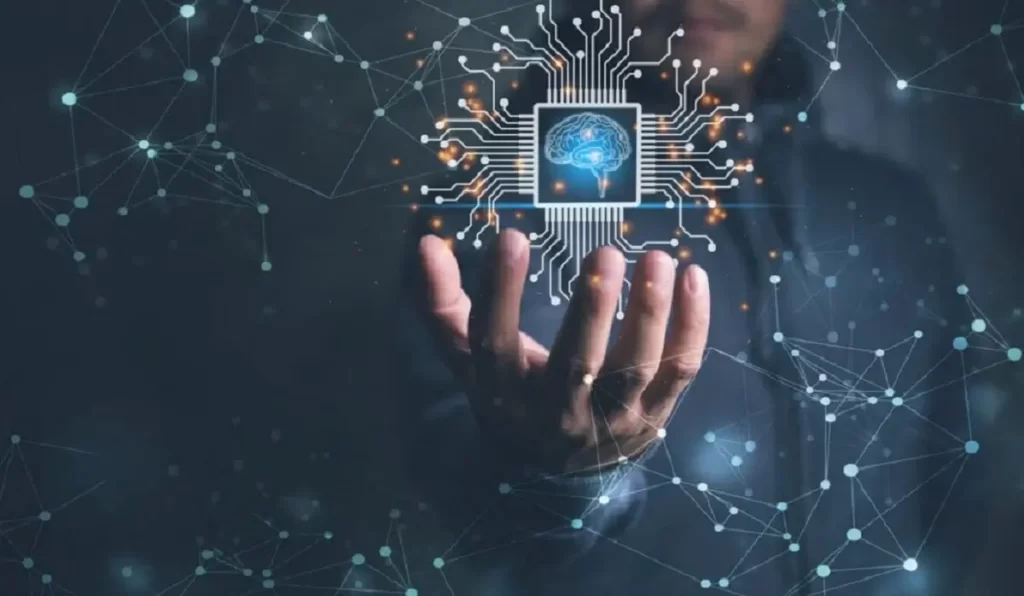
The choices made by developers and data scientists, such as which features to include in a model or how to weigh certain inputs, can introduce bias. Sometimes, even the choice of model can introduce bias.
Feedback Loops
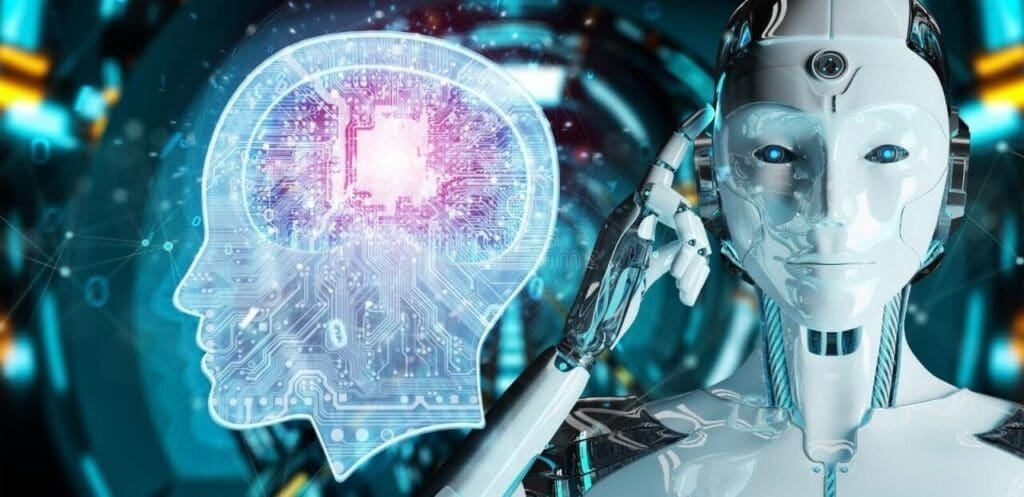
AI systems that adapt over time based on user feedback can get trapped in feedback loops. If the system initially exhibits a slight bias and users react to that biased output, the system can learn from this feedback and further entrench the bias.
Interpretability and Transparency Issues
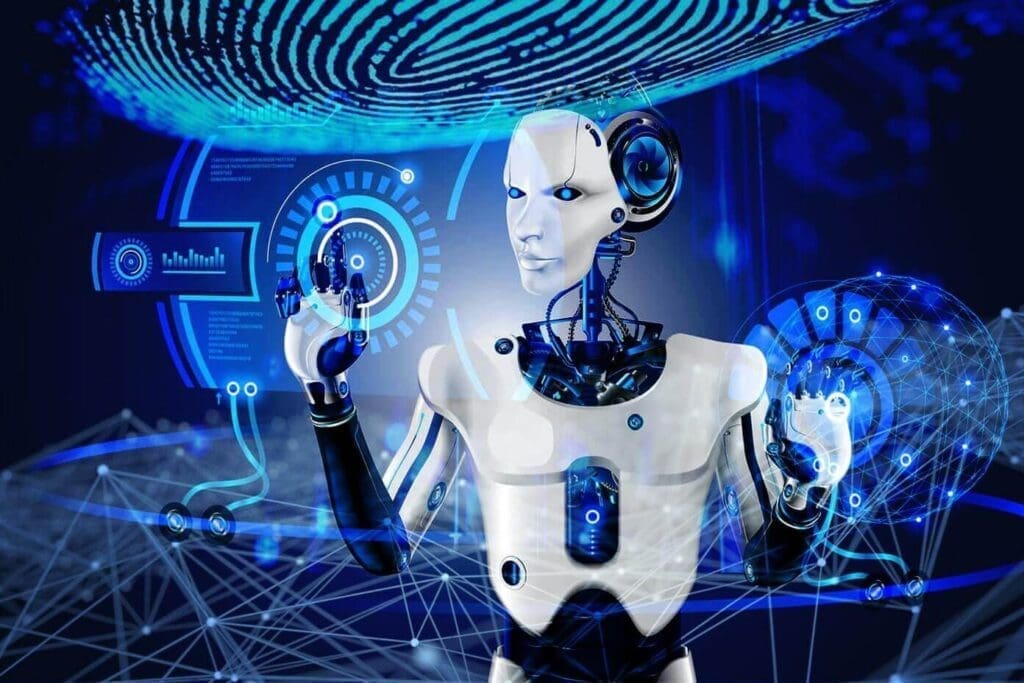
Many advanced machine learning models, like deep neural networks, are notoriously difficult to interpret. This lack of transparency can make it challenging to identify and correct biases.
Anthropomorphic Bias

Sometimes, AI system designers might embed human-like traits or societal norms into AI systems, whether intentionally or not, leading to biases.
Economic and Utility Biases
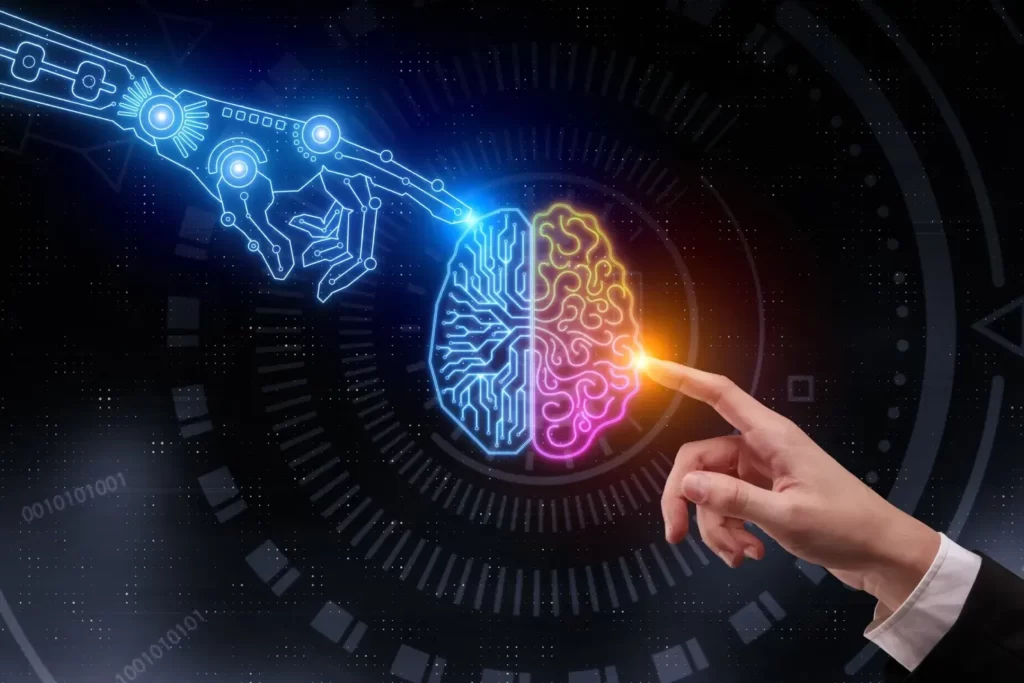
Sometimes, biases can be introduced when optimizing for economic outcomes or utility. For instance, an advertisement algorithm might consistently show high-paying job ads to one gender over another because historically that gender clicked on such ads more frequently.
Recognizing the potential for bias in AI is essential because biased AI systems can lead to unfair or discriminatory outcomes. The AI community is actively working on methods to detect, measure, and mitigate biases in AI models, but it remains a complex challenge. This awareness has led to a greater emphasis on ethical AI, fairness in machine learning, and the importance of diversity in the teams that design and deploy AI systems.
You may also like this content
- Microsoft Teams to Overcome Language Barriers with AI Translator Feature
- Discover Unique Artificial Intelligence Sites That Make Life Easier
- AI in Education: Transforming Learning Experiences Today
Follow us on TWITTER (X) and be instantly informed about the latest developments…