
Seeing the increasing danger from extreme risks requires a longer-term approach to address these threats for the future.
Big picture: The world was caught unprepared by COVID-19, and millions paid the price. However, the pandemic offers an opportunity to rethink our approach to low-probability, high-impact risks, including those we may inadvertently cause.
In the news: Earlier this week, the UK-based Nonprofit Long-Term Resilience Center released a report that global leaders need to read.
Led by Toby Ord, an existential risk expert at Oxford University, “Proof of Future” asserts that “we are currently living with an unsustainable level of extreme risk.”
As the authors write, “With the continuous acceleration of technology and without serious efforts to increase our resilience against these risks, there are strong reasons to believe that the risks will only continue to grow.”
Between the lines: “Proof of Future” focuses on two main areas of concern: artificial intelligence and biosecurity.
The long-term threat of artificial intelligence reaching superintelligence levels is an existential risk in itself. However, the proliferation of ransomware and other cyberattacks could be enhanced by AI tools, while the development of lethal autonomous weapons poses a threat and could make wars much more chaotic and destructive.
Natural pandemics are bad enough, but we are moving towards a world where thousands can access technologies capable of enhancing existing viruses or synthesizing entirely new ones. This point is far more dangerous.
It is still unclear how the world can control these human-made extreme risks.
Compared to nuclear weapons, the risks are more predictable – it is more difficult for a nation to build and use bombs without ensuring its own destruction, which is a major reason why fewer than ten countries have developed a nuclear arsenal 75 years after Hiroshima.
However, both biotechnology and artificial intelligence are dual-use technologies, meaning they can be used for both beneficial and malicious purposes. This makes them much harder to control than nuclear weapons, especially since some of the most extreme risks, such as a dangerous virus leaking from a lab, could be accidental rather than intentional.
Although the risks from biotechnology and artificial intelligence are increasing, little progress has been made in developing international agreements to manage them. While the UN office tasked with implementing the biological weapons ban has only three staff members, efforts to establish global norms around AI research – mostly conducted by private companies, unlike most in the nuclear field – have largely failed.
What to watch: The “Proof of Future” report recommends a range of actions from developing technologies like metagenomic sequencing, which can rapidly identify new pathogens, to countries allocating a percentage of their GDP to preparedness for extreme risks, much like NATO members are required to spend on defense.
A global agreement modeling previous efforts on nuclear weapons and climate change could at least elevate the international profile of extreme risks.
Most importantly, the report calls for the creation of “chief risk officers,” officials with the authority to review government policy with potential downsides in mind.
Conclusion: We are entering a daunting period for humanity. Ord estimates that within the next 100 years, there is a one-in-six chance of experiencing an existential catastrophe, equivalent to playing Russian roulette with our future.
If our actions have loaded the gun, it is also within our power to unload it.
Artificial Intelligence Work Areas , A Comprehensive Overview
AGRICULTURE
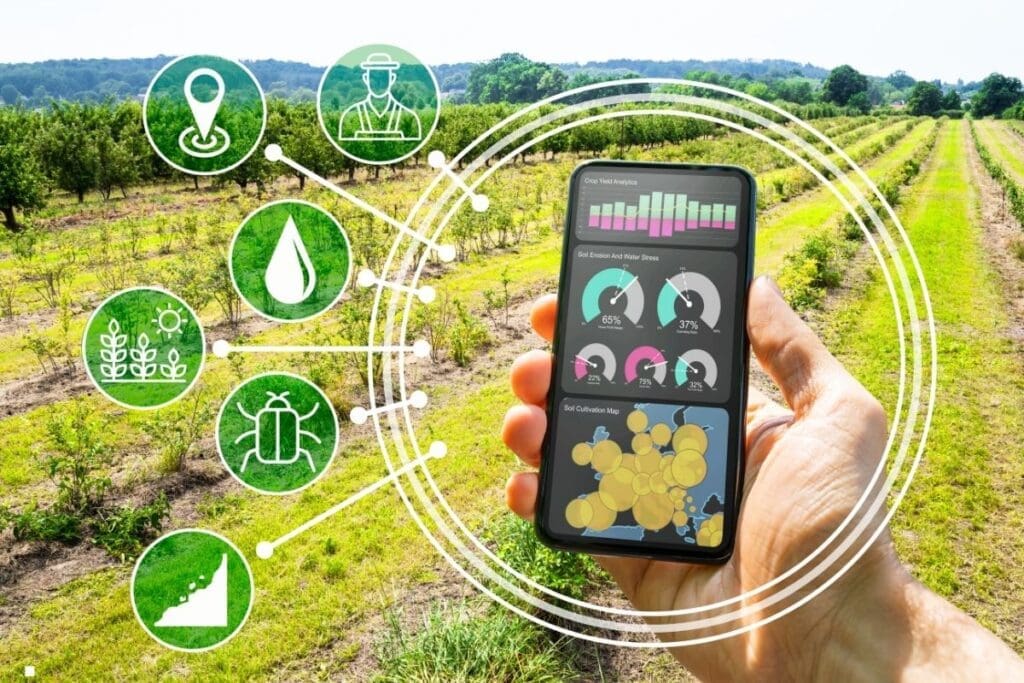
Agriculture and farming are among the world’s oldest and most crucial professions. Over thousands of years, humanity has evolved the way we plant and cultivate crops through the introduction of various technologies. As the global population continues to grow and land becomes scarcer, people need to be creative and more efficient in farming with less land while increasing the productivity and yield of the cultivated acres. Globally, agriculture is a $5 trillion industry now turning to AI technologies to achieve healthier crops, control pests, monitor soil and growing conditions, organize data for farmers, assist in workload management, and enhance diverse agricultural practices.
Farms generate hundreds of thousands of data points daily. With the help of artificial intelligence, farmers can now analyze various factors like weather conditions, temperature, water usage, or soil conditions from their farms in real-time. For instance, AI technologies help farmers optimize their planning for higher yields by determining the best crop choices, hybrid seed selections, and resource utilization.
AI systems also assist in improving harvest quality and accuracy, known as precision agriculture. This involves using AI technology to detect diseases, pests, and nutritional deficiencies in crops. AI sensors can identify and target weeds, then decide which herbicides to apply within the correct buffer zones. This helps prevent the over-application of herbicides and toxins finding their way into our food.
Farmers are also using AI to create seasonal forecast models to improve agricultural accuracy and boost productivity. These models can predict future weather patterns months in advance, aiding farmers’ decision-making. Seasonal forecasts are particularly valuable for small farms in developing countries, where data and resources may be limited. Keeping these small farms operational and productive is crucial, as they produce 70% of the world’s crops.
In addition to land data, farmers are looking to the skies to monitor their farms. Computer vision and deep learning algorithms process data collected by drones flying over fields. AI-enabled cameras can capture images of the entire farm from drones and analyze the images almost in real-time to identify problem areas and potential improvements. Unmanned drones allow for more frequent monitoring of large farms, covering much more land in much less time than humans.
AI is addressing the labor issue in farming
With fewer people entering the farming profession, many farms face a labor shortage problem. Traditionally, farms have required many workers, mostly seasonal, to harvest crops and keep the farms productive. However, as we move away from being an agrarian society with large populations living on farms, fewer people are inclined and available to work the land, now residing in cities. A solution to this labor shortage is AI agricultural bots. These bots enhance human labor efforts and are used in various ways. They can harvest crops at a higher volume and faster pace than human workers, more accurately identify and eliminate weeds, and provide a workforce at all hours, reducing farm costs.
Additionally, farmers are beginning to turn to chatbots for help. Chatbots assist by answering various questions and offering advice and recommendations on specific farm issues. Chatbots have already been successfully used in numerous other sectors.
Thanks to the use of artificial intelligence and cognitive technologies, farms worldwide can operate more efficiently with fewer workers while continuing to meet the world’s food needs. There is no more fundamental need than the need for food, and this will never disappear. Fortunately, the use of AI will enable farms of all sizes that feed our world to operate. With the use of agricultural AI and cognitive technologies, farms around the world can work more efficiently to produce the staple food items of our dietary lifestyles.
FINANCIAL SERVICES
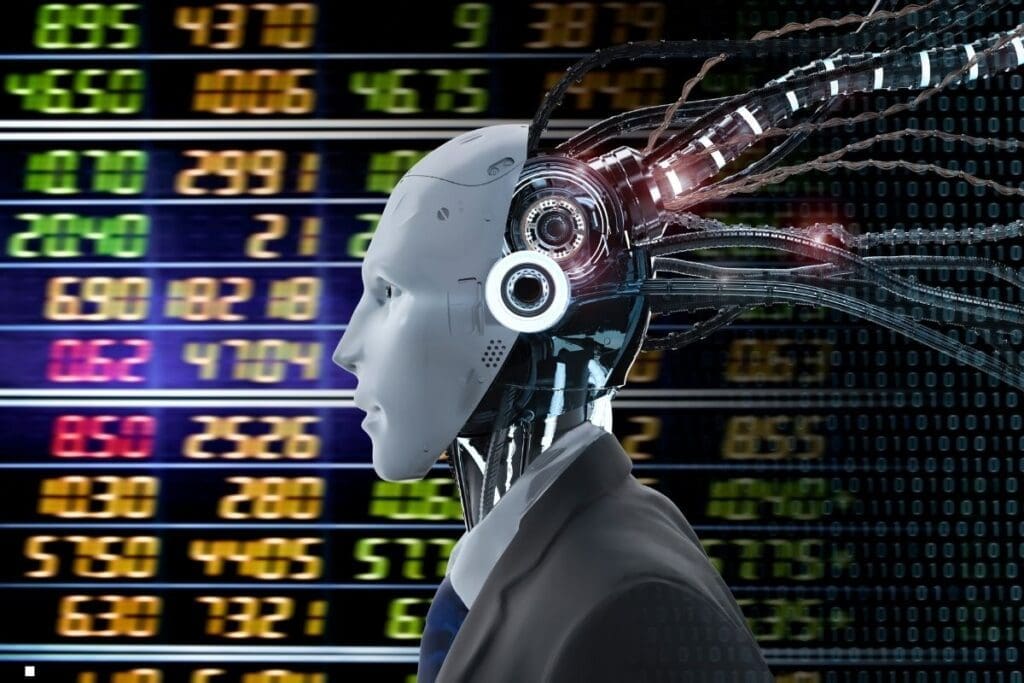
Like many other technological advances, Artificial Intelligence entered our lives straight from the pages of fantasy and fiction books: think of the Tinman from The Wizard of Oz or Maria from Metropolis. People dreamed of machines that could solve problems and alleviate some of the mounting pressures of the 21st century.
Less than 70 years after the term “Artificial Intelligence” was coined, AI has become an integral part of the most challenging and fast-paced industries. Forward-thinking executives and business owners actively explore new uses of AI in finance and other areas to gain a competitive edge in the marketplace. Often, we don’t realize how much AI has permeated our daily lives.
Where and Why AI Works Today
For instance, in the travel industry, AI helps optimize sales and pricing while also aiding in the prevention of fraudulent transactions. AI also enables personalized recommendations for desired dates, routes, and costs while planning our next summer vacation, navigating through airline or hotel booking sites.
In the transportation industry, AI is actively used in the development of advanced cruise control features that make self-parking and driving safer and easier.
Another shining example of AI use is in education, where online open courses (MOOCs) like Coursera or Lynda have become increasingly popular each year, thanks to the rise of AI in education. Automated grading and self-taught online courses are now accessible to anyone with internet access—a critical point for many lives and careers.
AI is a lifesaver, and this is not a metaphor. Doctors use AI for everything from robotic surgeries to virtual nurse assistants and patient monitoring. AI assists in various administrative tasks such as image analysis and scheduling, helping reduce costly human labor and allowing healthcare staff to spend more time with patients.
AI’s Rise in the Finance Sector
The rise of AI in finance demonstrates how quickly it has transformed the business environment, even in traditional areas. Here are some popular examples of AI in finance:
AI and Credit Decisions
AI allows for a quicker, more accurate evaluation of a potential debtor at lower costs, incorporating a wider variety of factors leading to more informed, data-driven decisions. AI-provided credit scoring is based on more complex and nuanced rules than traditional credit scoring systems. It helps lenders differentiate between applicants with high default risks and those who are creditworthy but lack a comprehensive credit history.
AI and Risk Management
When it comes to risk management, we must mention the impact of AI in financial services. Its tremendous processing power allows for the handling of vast amounts of data in a short time, and cognitive computing helps manage both structured and unstructured data, a task too time-consuming for humans. Algorithms analyze histories of risk cases and identify early signs of potential future issues.
AI and Fraud Prevention
For several years, AI has been very successful in combating financial fraud, and with machine learning catching criminals, the future looks even brighter every year. AI is particularly effective in preventing credit card fraud, which has grown exponentially in recent years due to the increase in e-commerce and online transactions. Fraud detection systems analyze customer behaviors, locations, and purchasing habits, triggering a security mechanism when something seems amiss and conflicts with established spending patterns.
As we can see, the benefits of AI in financial services are numerous and hard to ignore. According to Forbes, 65% of senior financial management expect positive changes from the use of AI in financial services. This comes at a time when only a third of companies had taken steps to implement AI in their business processes by the end of 2018. Many continue to be cautious, fearing the time and expense such an endeavor would require, and there will be challenges in implementing AI in financial services.
However, technological progress cannot be avoided forever, and avoiding it now may prove more costly in the long run.
HEALTH

Experts are discussing and examining the promises and opportunities of artificial intelligence in medical sciences across various platforms.
The extraordinary capabilities of artificial intelligence, such as processing vast amounts of data, interpreting images, and detecting subtle patterns that even the most skilled human eyes miss, offer hope that technology will transform medicine. Realizing the full potential of this opportunity will require the joint efforts of experts in computer science, medicine, policy, mathematics, ethics, and many other fields.
This interdisciplinary approach was the focus of the discussions at the Artificial Intelligence and Imaging Center (AIMI) online conference held on August 5th. The event, which featured world experts from the fields of computer science, medicine, industry, and government, focused on discussions centered around emerging clinical machines, technological innovations, data ethics, policy, and regulation. The symposium was led by Matthew Lungren, Associate Professor of Radiology and Co-Director of AIMI, and Serena Yeung, Assistant Professor of Biomedical Data Science and Computer Science and Associate Director of AIMI.
Here are some notes from the day-long conference:
The Greatest Potential of AI
Eric Topol, a renowned cardiologist, researcher, and author from Scripps Research, sees three areas where artificial intelligence has the greatest potential to transform medicine:
- Reducing medical errors that lead to misdiagnosis. Recent studies show that AI-supported human pathology is more effective in detecting breast cancers, particularly in reducing false-negative mammograms that delay patient care.
- There will be numerous new applications, like smartphone apps that diagnose skin cancer, helping people manage their health throughout their lives. This will be known as the “medical selfie” era.
- AI can reduce or eliminate the drudgery of data entry that leads to doctor fatigue and steals valuable time from patients.
Democratizing Data
Simply creating medical AI products is not enough. It’s crucial to make these products accessible to people. Lily Peng, product manager at Google Brain Artificial Intelligence Research Group, said, “The world’s best product is useless if people can’t access it.” She highlighted a new model her team is working on, which can achieve similar results with a smaller, higher-quality data set and could potentially accelerate the market introduction of a valuable product. “We need to bring these products closer to people,” she added.
Stanford researcher Pranav Rajpurkar noted that algorithms trained on proprietary or incomplete data sets tend to fail outside these friendly confines – they do not generalize well. For instance, AI models trained on lung diseases in the American datasets, which do not include tuberculosis screenings, fail to detect tuberculosis, a significant issue in the developing world. True democratization requires AI to work everywhere for everyone. Simply adding tuberculosis images to American training datasets would help generalize and democratize valuable artificial intelligence to other regions of the world.
Gilberto Szarf, a chest radiologist from Brazil, discussed that democratization in his home country means using artificial intelligence to provide or accelerate care in places where resources and expertise are scarce for conditions like melanoma, tuberculosis, Zika, and even COVID-19. An AI model for diagnosing Zika from medical images would be a valuable tool in regions of Brazil where quality medical care is difficult to access.
NATIONAL SECURITY AND DEFENCE INDUSTRY
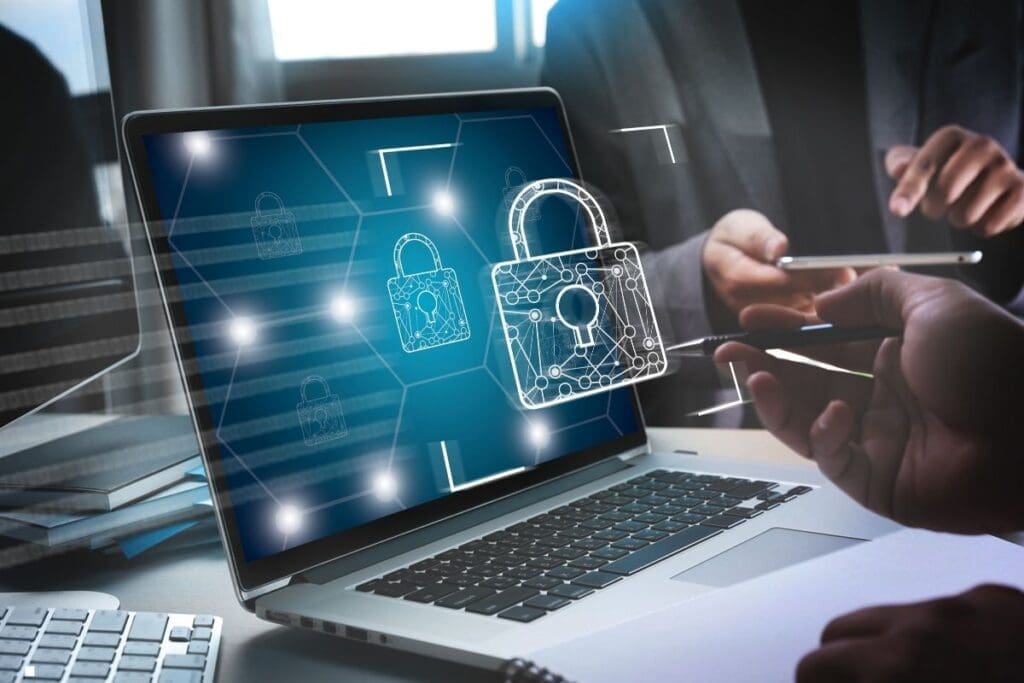
Artificial Intelligence (AI) is a rapidly growing field with potentially significant impacts on national security. Consequently, Turkey and other countries are developing AI applications for a variety of military functions. Ongoing AI research spans intelligence gathering and analysis, logistics, cyber operations, information operations, command and control, and various semi-autonomous and autonomous vehicles. Budget and legislative decisions, which influence the growth and adoption rate of military applications, have the potential to further shape the development of technology. AI technologies pose unique challenges for military integration, particularly since much of AI development occurs in the commercial sector. While not unique in this regard, the defense acquisition process may need to be adapted to acquire emerging technologies like AI.
Additionally, many commercial AI applications must undergo significant modifications before they can be functional for military use. Some commercial AI companies are reluctant to partner with the Department of Defense (DOD) due to ethical concerns, and there may be resistance within the department itself to integrating AI technology into existing weapon systems and processes. Potential international competitors in the AI market create pressure for the United States to compete in innovative military AI applications. China is a leading competitor in this area and published a plan in 2017 aiming to achieve global leadership in AI development by 2030. Currently, China is focusing on using AI to make faster and more informed decisions and also on developing various autonomous military vehicles. Russia is also active in military AI development, primarily focusing on robotics. While AI has the potential to provide a range of advantages in a military context, it can also introduce various challenges. For example, AI technology could facilitate autonomous operations, lead to more informed military decision-making, and increase the speed and scale of military operations. However, it might be vulnerable to unpredictable or unique forms of manipulation. As a result, analysts have a wide range of views on how effective AI will be in future combat operations. While a few analysts believe the technology will have minimal impact, most believe that AI will at least have an evolutionary (if not revolutionary) effect. Military AI development presents a series of potential issues for Congress:
What is the right balance between commercial and government funding for AI development?
How might defense acquisition reform initiatives that facilitate military AI development be influenced?
What changes are necessary in Congress and the Department of Defense to implement effective oversight of AI development?
How should the United States balance research and development related to AI and autonomous systems with ethical concerns?
What legal or regulatory changes are required for the integration of military AI applications?
What measures can Congress take to help manage global competition in AI?
SCIENCE
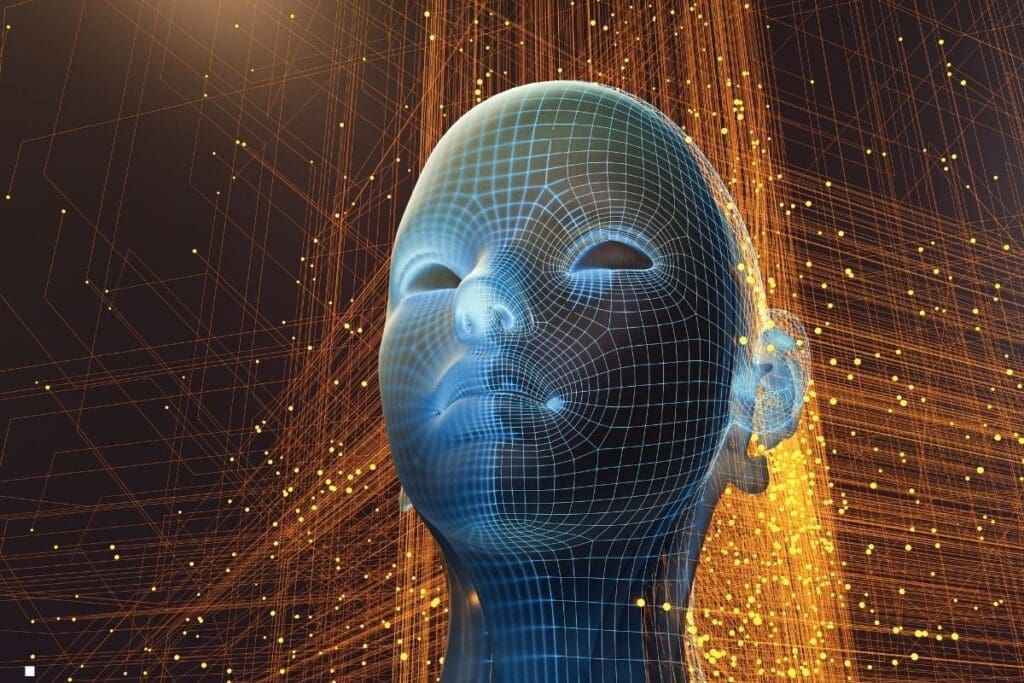
Artificial intelligence (AI) technologies are now employed across various scientific research fields. For instance:
Using Genomic Data to Predict Protein Structures
Understanding the shape of a protein is key to comprehending its role in the body. Scientists can identify proteins involved in diseases by predicting their structures, which enhances diagnosis and aids in developing new treatments. Determining protein structures is both technically challenging and labor-intensive, and to date, only about 100,000 known structures have been identified. Recent advancements in genetics provide rich DNA sequence datasets, while determining a protein’s shape from its corresponding genetic sequence (the protein folding challenge) remains complex. Researchers are developing machine learning approaches that predict the three-dimensional structure of proteins from DNA sequences. For example, DeepMind’s AlphaFold project has created a deep neural network that predicts distances between amino acid pairs and the angles between bonds, accurately estimating the overall structure of a protein.
Understanding the Impacts of Climate Change on Cities and Regions
Environmental science combines the need to analyze large amounts of recorded data with complex system modeling (as required to understand the impacts of climate change). To inform decision-making at national or local levels, it is necessary to interpret predictions from global climate models in terms of outcomes for cities or regions; for instance, predicting the number of days in a city where temperatures will exceed 30°C within 20 years. Local areas, such as those with detailed observational data from weather stations, can access detailed information about local environmental conditions, but making accurate predictions from these alone is challenging given the fundamental changes brought about by climate change. Machine learning can help bridge the gap between these two types of information. The low-resolution outputs of climate models can be integrated with detailed, local observational data. The resulting hybrid analysis will improve climate models created with traditional analytical methods and provide a more detailed picture of local effects of climate change. For example, a current research project at the University of Cambridge is exploring how climate variability in Egypt will change over the coming decades and how these changes will affect cotton production in the region. The resulting predictions can then be used to devise strategies to build climate resilience that will mitigate the impacts of climate change on regional agriculture.
Finding Patterns in Astronomical Data
Astronomical research generates vast amounts of data, and a major challenge is detecting interesting features or signals from the noise and correctly categorizing them. For instance, the Kepler mission aims to discover Earth-sized planets orbiting other stars, collecting data that may indicate the presence of stars or planets in the Orion Spur and beyond. However, not all these data are useful; they can be distorted by the activity of onboard thrusters, changes in star activity, or other systematic trends. Before the data can be analyzed, these so-called instrumental errors must be removed from the system. To assist, researchers have developed a machine learning system that can identify these noises and remove them from the system, subsequently cleaning the data for analysis. Machine learning has also been used to discover new astronomical phenomena, such as: finding new pulsars from existing datasets; determining the characteristics of stars and supernovae; and accurately classifying galaxies.
TRANSPORT

Artificial intelligence is transforming the transportation sector, from facilitating autonomous operation of cars, trains, ships, and airplanes to smoothing traffic flow across various transportation modes. Beyond making our lives easier, AI can help make all modes of transport safer, cleaner, smarter, and more efficient. AI-driven autonomous transportation, for example, can help reduce human errors, which are involved in many traffic accidents. However, with these opportunities come real challenges, including the potential for cyberattacks and biased decisions related to transportation. There are also employment and ethical questions related to decisions made by AI instead of humans.
The European Union is taking steps to adapt its regulatory framework to these developments, supporting innovation while ensuring respect for fundamental values and rights. Current measures include general strategies on artificial intelligence and rules that support technologies enabling the application of AI in transportation. The EU is also providing financial support, especially for research, to further these initiatives.
WEATHER FORECAST

Modern weather forecasting relies on a vast data collection network. The addition of high-resolution remote sensors provides a foundation for more accurate and precise weather predictions, but it also raises questions about how to process, understand, and maximize the use of all this data most effectively.
Traditionally, weather forecasting has focused on developing complex dynamic numerical models aimed at more accurate predictions. However, due to certain disadvantages such as the inherent uncertainty of weather and coordination among different models, this method may not meet the requirements of various use cases. To bridge this gap, artificial intelligence (AI) and data-driven methods have been introduced.
AI is not new to meteorology; it has found applications in weather forecasting since the 1980s when neural networks were first introduced. In recent years, as AI models have gained momentum in various industries, meteorology researchers are now applying this technology to satellite data processing, real-time forecasting, typhoon and extreme weather prediction, and other business and environmental analytics.
The journal Earth and Space Science describes AI technologies as key to providing more accurate and timely forecasts while reducing the workload of human forecasters. The U.S. National Oceanic and Atmospheric Administration (NOAA) also notes that integrating artificial intelligence and machine learning has significantly enhanced the capability to predict extreme weather conditions such as storms and hurricanes.
Google is an industry pioneer. In December 2019, the tech giant presented new research on developing deep learning models for precipitation forecasting. The team approached forecasting as an image-to-image translation problem, leveraging the power of the widely-used U-Net convolutional neural network. U-Net has a network architecture where the resolution of images is progressively reduced during an encoding phase, and the low-dimensional representations created by the encoding phase are then expanded to higher resolutions during a subsequent decoding phase. In tests, the proposed system outperformed three commonly used models: optical flow, persistence, and NOAA’s numerical one-hour HRRR nowcast.
Major companies are also forming partnerships to maintain the trend. In 2015, IBM acquired The Weather Company. The merger of technology and expertise in weather data from both companies has resulted in the Atmospheric Forecasting System, which offers a variety of forecast services including predictions of weather-related power outages up to 72 hours in advance, using machine learning models. The system is reported to be the first operational global weather model running on GPU-accelerated servers, designed to handle increased resolution and frequent updates.
In Shenzhen, China, the meteorological office is exploring ways to improve weather forecasts in the challenging Guangdong coastal region, known for severe convective weather. The office has collaborated with tech giant Huawei to create a meteorological cloud platform equipped with 5G and AI capabilities to accelerate the development, training, and deployment of forecast models from 1-2 weeks to 3 days or less.
Startups are also positioning themselves as game-changers in the industry. ClimaCell’s patented MicroWeather engine applies machine learning to historical gridded weather data to increase accuracy in weather forecasting. The company recently launched an historical weather data archive for training AI models using data collected from a global network of wireless signals, connected cars, airplanes, street cameras, drones, and other Internet of Things (IoT) devices.
While AI will continue to play a significant role in weather forecasting, attracting AI talent to the field has not been easy. Meteorological offices cannot compete with the salaries offered by technology companies focusing on glamorous sectors like autonomous vehicles and computer vision. Instead, we see technology giants partnering with local meteorological organizations or taking over the work themselves. Globally, we can expect an increase in such arrangements in the future.
INTERNATIONAL CO-OPERATIONS
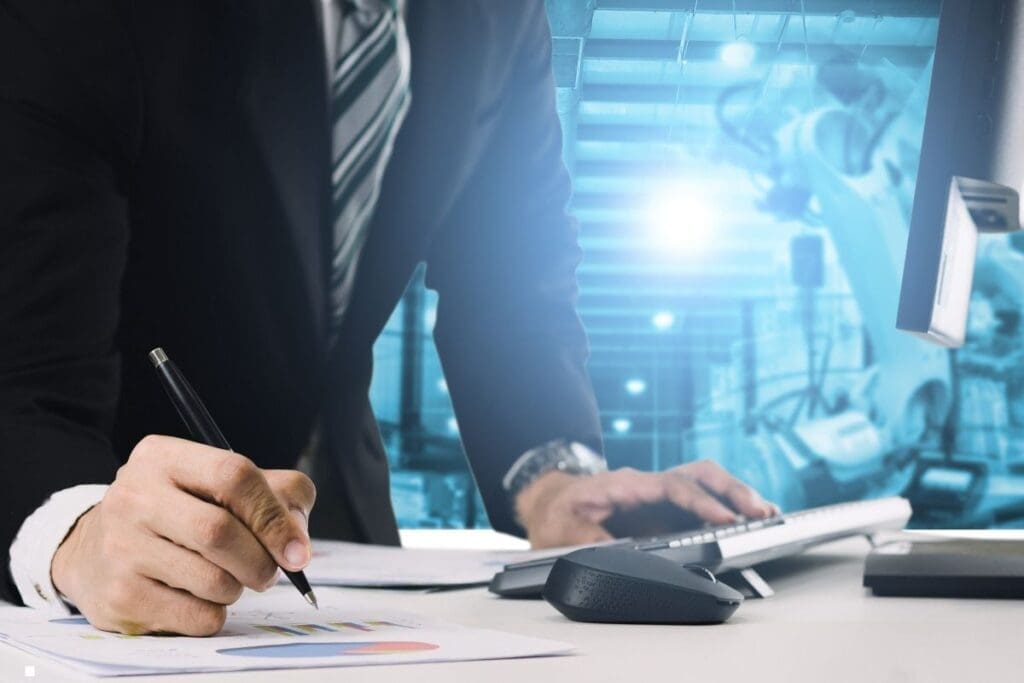
The facilitation of remote working methods by current technologies allows access to skilled workforces around the world. In addition, the collaborative use of domain experts, technical infrastructure, and data pools from different countries can lead to the development of more successful technologies and applications in the field of Artificial Intelligence (AI).
Furthermore, the development of AI technologies and the extensive collection of data necessary for this, often involving citizens from different countries, are causing tensions between major technology companies and countries possessing the data, bringing up discussions on digital sovereignty. Therefore, to manage the mentioned tensions and derive greater global benefits from AI technologies, there is a need to develop international collaborations in the field of Artificial Intelligence.
You may also like this content
- Top 1000 Sci-Fi Movies About Metaverse, AI, and Science
- How to Use iPhones’ New Emoji Creation Feature “Genmoji”
- OpenAI Introduces Sora: A Revolutionary Text-to-Video Creation Tool
Follow us on TWITTER (X) and be instantly informed about the latest developments…